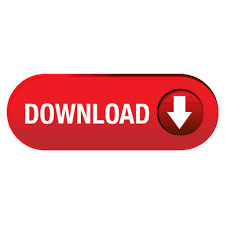

We’ve never really unbundled those aspects of decision making before-we usually think of human decision making as a single step. We use both prediction and judgment to make decisions. But there are other complements to prediction that have been discussed a lot less frequently. The very interesting twist is here, where he mentions the trope of “data is the new oil” but instead presents judgment as the other complement which will gain in value. Its predictions start getting better and better until it becomes so good at predicting what a human would do that we don’t need the human to do it anymore. But it learns from its mistakes and updates its model every time it incorrectly predicts an action the human will take. … The AI makes a lot of mistakes at first. He then looks at AI and frames it around the reduction of the cost of prediction, first showing how AIs lower the value of our own predictions. The value of these increased because we used more of them, while the value of substitutes, the components of film-based cameras, went down because we started using less and less of them.
GRIM DAWN MAP SHOWING UNRESTORED SHRINE SOFTWARE
So, in the case of photography, the complements were the software and hardware used in digital cameras. The third thing that happened as the cost of arithmetic fell was that it changed the value of other things-the value of arithmetic’s complements went up and the value of its substitutes went down.

It starts with the example of the microprocessor, an invention he frames as “reducing the cost of arithmetic.” He then presents the impact as lowering the cost of the substitute and raising the value of the complements. This Mckinsey piece summarizes some of Ajay Agrawal thinking (and book) on the economics of artificial intelligence. Results show a 30% decrease in navigation path lengths, 24% decrease in task time, 15% decrease in mental demand and 29% decrease in frustration in favor of egocentric navigation. We present a study on haptic search tasks comparing spatial manipulation of a shape display for egocentric exploration of a map versus exploration using a fixed display and a touch pad. shapeShift can also be mounted on an omnidirectional-robot to provide both vertical and lateral kinesthetic feedback, display moving objects, or act as an encountered-type haptic device for VR. shapeShift can be mounted on passive rollers allowing for bimanual interaction where the user can freely manipulate the system while it renders spatially relevant content. To explore these interactions, we developed shapeShift, a compact, high-resolution (7 mm pitch), mobile tabletop shape display. We explore interactions enabled by 2D spatial manipulation and self-actuation of a tabletop shape display. We’ve seen this before with the MIT Media Lab’s Tangible Media Group inFORM but that was a (super impressive) table, this one is movable, much smaller, seems to be higher resolution and multiple units can be combined.
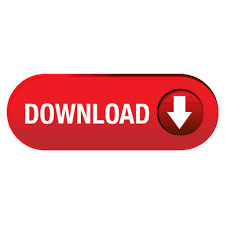